Over the last two years, the increasing complexity of modern distributed systems and application architectures has expanded the security surface area of every enterprise. It has also highlighted the limits of legacy detection and response approaches. Detection and response tooling, like XDR and SIEM, remains fixated on collecting and reporting alerts, burying teams in low-value work doing triage and potentially missing critical breaches. Applying observability principles to today’s security environments allows teams to boost the signal in a sea of noisy data and see more of what’s happening across their environments, without the limits imposed by legacy methods and products.
What is Observability?
Observability comes from industrial control theory in the 1960s. The idea is, that if you have enough data, you can infer the internal state of a system from its outputs. This takes a fundamentally different approach than monitoring’s alert-based methods; it allows you to ask questions about a system that digs deeper than the pre-defined thresholds from your monitoring and alerting systems. Observability isn’t a replacement for monitoring, however, each plays a role. The reality is you need both observability and monitoring for full insight into your environment. Observability gives you greater flexibility for coping with unknowns, while monitoring offers reliable processes for dealing with the expected.
The Challenge of Processing so Much Data
Observability requires collecting massive amounts of data from systems, networks, and applications to feed its discovery process. As systems and applications become more complex, figuring out what went wrong and why becomes much more challenging. Collecting logs, metrics, events, and traces from different sources such as firewalls, agents, containers, and syslog servers, then sending these huge volumes of data to various destinations such as your SIEM tools or data analytics platforms can create an unnecessary financial and technical burden on your organization’s infrastructure. Most enterprises have 20 to 30 monitoring tools, each with its own dedicated agents, resulting in disconnected silos of information. With increasing demands to retain and monitor all forms of data traversing a company’s network, security cannot simply discard excess data, so the path forward becomes streamlining data processing.
Observability Pipelines
Coping with this data challenge requires a new way of thinking about observability data. Companies are adopting specially-designed pipelines to connect the sources and destinations of observability and security data. These pipelines allow companies to route data to multiple destinations, enrich data in flight and reduce data volumes before ingestion. The term ‘observability pipeline’ addresses a very specific problem that arises from data and tool sprawl.
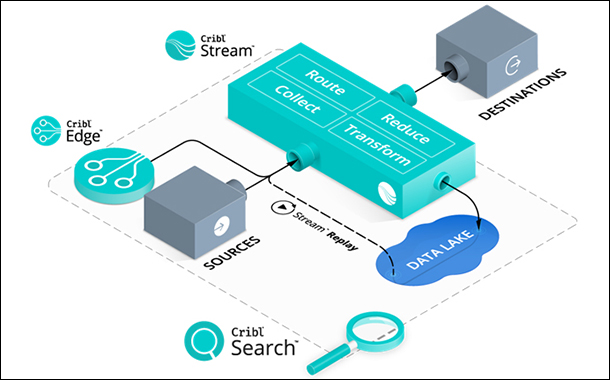
An observability pipeline is a strategic control layer positioned between the various sources of data, like networks, servers, applications, and software agents, and the multiple destinations in today’s IT and SecOps environments. Instead of relying on siloed point-to-point connections, an observability pipeline centralizes all of your observability data processing, giving your teams full control over every aspect of your data.
Abstracting the sources and destinations of observability data offers massive benefits to IT and SecOps teams. For example, it can provide a single point for governing data and applying consistent rules for data redaction, access control, and sharing. It can also help reduce the amount of redundant data flowing into downstream systems like logging analytics, SIEM, and SOAR platforms, or accelerate the onboarding of new tools by sharing data from one source with multiple destinations.
The observability market is maturing whilst continually opening new doors for opportunities and growth of data management and insight. As the amount of data and toolsets increase, organizations want to keep an eye on the management and complexity involved in architecting and orchestrating at a continually increasing scale.